When the Metric Becomes the Monster
A dive into Goodhart’s Law and how smart teams accidentally optimize for the wrong thing.
Fellow Data Tinkerers!
As I mentioned earlier this week, you can have access to 100+ cheat sheets covering everything from Python, SQL, Spark to Power BI, Tableau, Git and many more. You just need to share Data Tinkerer with 2 other people to unlock it
So if you know other people who like staying up to date on all things data, please share Data Tinkerer with them!
Now, without further ado, let’s get into a fallacy you want to avoid!
At some point in every org’s journey toward being “data-driven,” someone has a bright idea:
“Let’s pick a North Star metric and optimise everything around it.”
It sounds reasonable. Smart, even.
Until three months later, when you realise your customer satisfaction score is going up, but actual customer satisfaction is not. Or support ticket resolution time is down because agents are closing tickets before reading them.
What happened?
You’ve just met Goodhart’s Law:
When a measure becomes a target, it ceases to be a good measure.
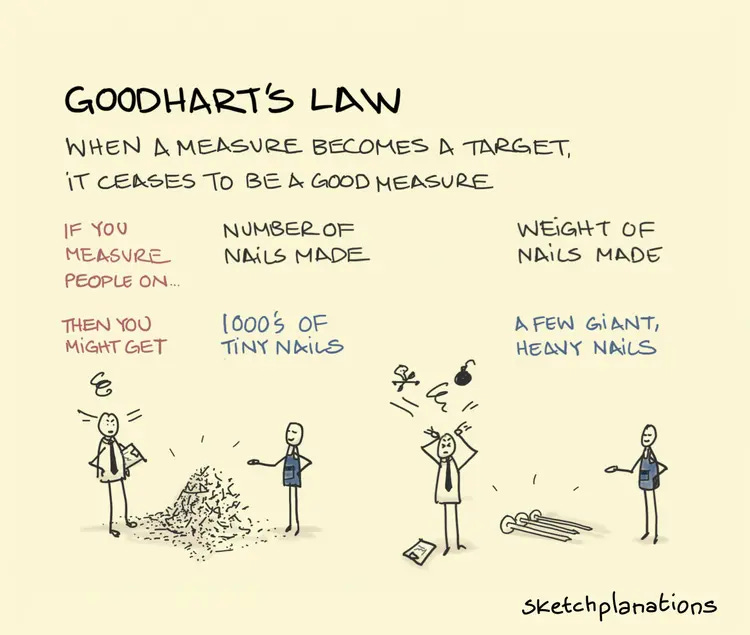
The spirit of the metric vs. the letter of the metric
Most metrics start with good intentions.
Time to resolve = help customers quickly
Daily active users = make something people want
Click-through rate = are we getting attention?
But once those metrics become goals tied to incentives, visibility, or OKRs, something shifts.
The goal stops being improve the experience and becomes get the number to move.
That’s when people start:
Chasing edge cases
Gaming definitions
Doing things that hit the number but miss the point
Real-world examples (that might have happened in your company)
Support:
Resolution time becomes the KPI.
Support staff rush to close tickets. And cause the issue is not completely resolved, the customers re-open them. But hey, first response time looked great.
Marketing:
Click-through rate is the target.
Campaigns turn into clickbait, engagement drops and unsubscribes go up. But the CTR? Beautiful.
Product:
Daily active users is king.
You start nudging people back into the app with notifications they didn’t ask for. They open it… and close it.
Technically active. Emotionally gone.
Sales:
Demo calls booked becomes the metric.
Reps start qualifying anyone with a phone number. Close rates tank. But calendar invites are full.
Why Goodhart’s Law happens
Humans are clever.
Give them a rule and they’ll find a way to follow it in the letter but not the spirit, especially when rewards or performance reviews are involved.
As Charlie Munger used to say it often:
“Show me the incentive and I will show you the outcome.”
What makes it tricky is that the metric still moves. It just doesn’t mean what you think it means anymore.
That’s the trap.
But how to avoid being gamed by your own metrics then?
To be perfectly honest, you can’t totally prevent it but you can design around it:
1. Pair metrics with context
Don’t just track one number. Track a set of counterbalancing metrics.
Time to resolution? Pair it with customer satisfaction.
CTR? Track bounce rate too.
2. Rotate your metrics occasionally
What works this quarter might be gamed by next quarter.
Metrics need freshness. Or at least revalidation.
3. Watch for side effects
If a metric improves but the user experience worsens, dig deeper.
What got optimised and what got ignored instead?
4. Ask teams “how” not just “how much”
When someone hits their goal, ask how they got there. If the answer makes you wince, your metric is broken.
5. Reward outcomes, not activity
Shift focus from hitting the number to solving the problem. The best metric is the one no one is trying to game because they don’t need to.
Goodhart’s Law isn’t about bad people or bad intentions. It’s about the natural outcome of treating metrics like strategy.
Metrics are supposed to be signals, not scoreboards. When they become the scoreboard, everyone starts playing a different game.
So yes, measure what matters. Just keep checking that what you’re measuring still does matter.
Really insightful read! hHow do you think organizations can best balance the need for measurable goals without falling into the Goodhart’s Law trap? Is it more about refining metrics continuously, or should we rethink our reliance on metrics altogether?